Top Data Science Trends Transforming the Industry in 2025
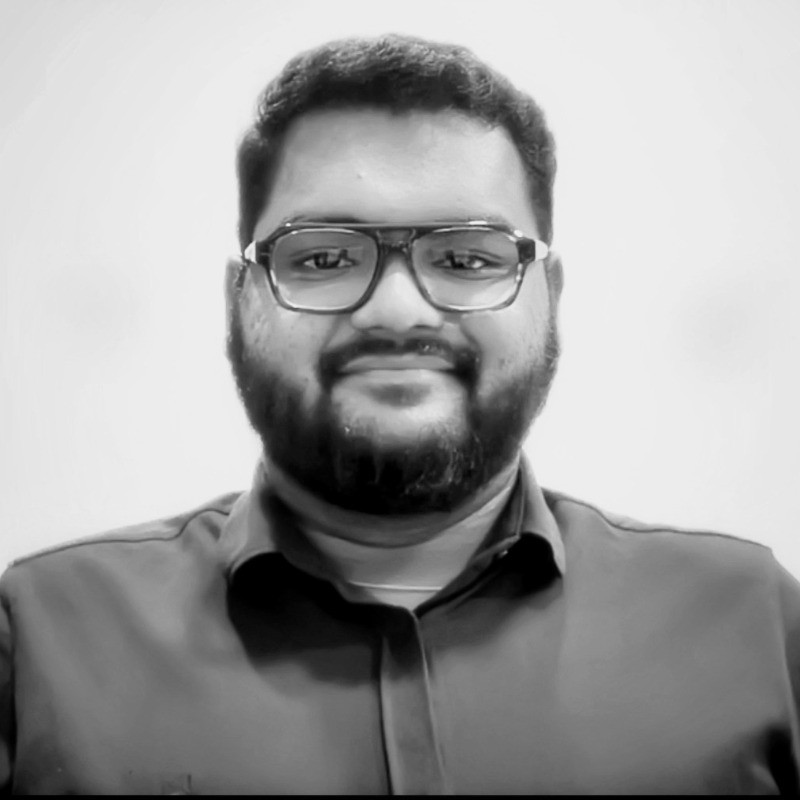
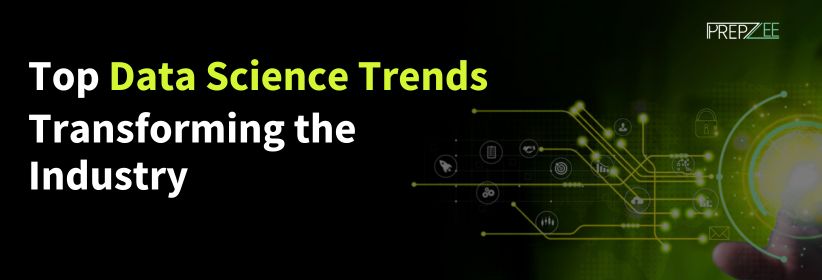
Table of content
As we move along in 2025, the world of data science keeps changing at a very fast pace with technology and changing business needs. To stay ahead for professionals and companies, it is crucial to keep track of these new trends. Data science has become very popular because it is multidisciplinary and effective. With the integration of artificial intelligence (AI) and machine learning (ML), data science is revolutionizing innovation and revolutionizing industries globally. This article delves into the newest trends that are defining the future of data science in 2025 and beyond, providing insights for beginners and experts alike. Data science has become a very sophisticated field, combining human ingenuity with AI and ML to improve decision-making and automate intricate processes. Its uses range from predictive analytics, big data processing, and intelligent automation to improving efficiency and accuracy across sectors.
Here’s a comprehensive exploration of the top data science trends reshaping the industry this year.
Top Data Science Trends
1. Augmented Analytics: Enabling Decision Intelligence
Augmented analytics utilizes machine learning (ML) and artificial intelligence (AI) to make data analysis easier. This trend enables analytics to reach more people, and business users without sophisticated technical knowledge can now make informed decisions. This allows organizations to act more rapidly and be more innovative.
2. Natural Language Processing (NLP): Human-Machine Communication
Advances in NLP are enabling computers to interpret and generate human language. This enables humans to naturally interact more with computers, enhancing applications such as chatbots, virtual assistants, and sentiment analysis. Because of this, companies can create more personalized and streamlined customer experiences.
3. Automated Machine Learning (AutoML): Democratizing Model Development
AutoML simplifies machine learning model construction through the automation of activities like data preparation, feature selection, and tuning the model. This simplicity allows individuals who have minimal ML experience to build good models, and it makes machine learning more accessible and promotes new innovation across various disciplines.
4. Edge Computing: Computing Data in Real-Time at the Source
Edge computing and the Internet of Things (IoT) are revolutionizing the way we process information by enabling real-time processing where data is created. The approach reduces latency and bandwidth consumption, which is critical for applications requiring information in real time, such as autonomous vehicles and industrial automation.
5. Synthetic Data Generation: Dealing with Data Sparsity
The need for immense data to train AI models has encouraged the use of synthetic data generation techniques. Corporates like Nvidia are funding synthetic data companies to generate large datasets, especially where real data is hard to find or is confidential. Synthetic data has the ability to scale and preserve privacy but needs to be combined with real-world data to keep the models up to date and reliable.
6. Quantum Computing: Solving Hard Problems
Quantum computing is increasingly becoming a vital technology that can crack problems that are unsolvable on regular computers. In data science, quantum algorithms have the potential to revolutionize how we optimize, how we encrypt and decrypt, and how we simulate materials. Despite the fact that it is in its infancy, research and development indicate a promising future for quantum technology in data analytics courses.
7. Explainable AI (XAI): Establishing Trust and Transparency
With more advanced AI models being developed, understanding how they operate is crucial. Explainable AI (XAI) makes this possible by depicting how models make decisions, hence building trust among stakeholders. Fields such as healthcare and finance, where decision-making is extremely critical, especially stand to gain from XAI, allowing for regulation and ethical compliance.
8. Safeguarding Information and Privacy: Complying with Regulations and Building Trust
As data breaches are becoming more frequent and regulations such as GDPR become more stringent, organizations are paying attention to data privacy and security. Techniques such as differential privacy and federated learning are gaining traction, enabling data analysis without compromising personal privacy. These techniques are crucial to maintaining customer trust and preventing legal trouble.
9. Real-Time Analytics: Instant Insights for Quick Decision-Making
The need for real-time decision-making has been the motivation for the implementation of real-time analytics. Firms use streaming data to track operations, identify anomalies, and respond quickly to shifting conditions. The ability is crucial in finance, healthcare, and e-commerce industries where timely information can translate into a competitive advantage.
10. Data Ethics: Maintaining Integrity in Data Science
As data-driven decisions affect increasingly more aspects of society, ethics become increasingly relevant. Data scientists increasingly have an obligation to ensure that their models are responsible, fair, and transparent. Building ethical practices and standards is vital to prevent bias and preserve the credibility of data science implementations.
11. AI for Weather Forecast: Improving Accuracies
AI techniques are revolutionizing the way we predict the weather by making the predictions sooner and more accurately using less computing power. For instance, the Aardvark Weather system employs AI trained on raw data from weather sources globally, which enables precise predictions on ordinary desktop computers. This is an enhancement that makes forecasting more accessible to everyone, particularly in areas with limited access to powerful computers.
12. DNA Data Storage: New Concepts for Data Preservation
The exponential increase in digital data has led scientists to explore DNA as a storage device for data. DNA storage allows for long-term, high-density storage, and this could potentially change the means of storing and retrieving information. Microsoft and Catalog are among the top companies that synthesize and store data in DNA, with the aim of addressing data preservation and data management challenges.
13. Agentic AI Systems: Autonomous Decision-Making
Agentic AI is systems that can act independently and make decisions. Agentic AI agents can conduct tasks independently without any intervention from humans, presenting new avenues for automation and streamlining in most sectors. Organisations that want to harness the power of AI to the fullest must learn to cooperate with agentic AI.
14. Small Data and Few-Shot Learning
While big data remains the focus of interest, interest in small data and few-shot learning techniques is also growing. They enable models to learn in an efficient manner from small sets of data, eradicating the necessity of huge data sets of labels. Few-shot learning techniques, catalyzed by the advances in transfer learning and deep learning, become particularly beneficial for applications in which data collection is expensive or difficult. Healthcare, among other areas of study in which there is no abundant quantity of labeled medical data, is greatly aided by these techniques.
15. Multimodal AI: Integrating Complementary Sources of Information
Multimodal AI enhances machine learning by pulling different types of data—text, images, sound, and video—into one framework. This enables AI to learn more and create more integrated models, making applications like medical diagnosis, self-driving cars, and content recommendation systems improved. OpenAI and Google DeepMind are refining multimodal AI to enable AI to comprehend more and interact more.
16. AI-Powered Software Development: Simplifying Coding
AI technologies such as GitHub Copilot and OpenAI Codex are revolutionizing software development as they automate processes such as writing code, debugging, and optimizing code for performance. AI assistants enable developers to write improved code in a shorter time, thus reducing development time and improving software quality. As AI gains popularity in software engineering, businesses are finding more efficiency and productivity in their development.
17. Leveraging AI for Hyper-Personalization
Hyper-personalization enabled by AI is transforming marketing, e-commerce, and how businesses interact with customers by offering them highly personalized content and suggestions. With real-time behavior data, machine learning can make precise forecasts of what consumers desire, leading to higher sales and better customer satisfaction. Amazon and Netflix are leading the way by using AI to power recommendation engines and make customer experiences better.
18. AI in Cybersecurity: Threat Detection in Advance
With the growing cyber threats, AI is a significantly important aspect of cybersecurity because it identifies unusual patterns, detects potential threats, and provides automated responses to threats. AI-powered security products scan gigantic amounts of network traffic in real-time, keeping organizations one step ahead of cyber attackers. Advanced technologies such as behavioral analytics and AI-powered SIEM systems have become a vital component of cybersecurity solutions in today’s era.
19. Artificial Intelligence in Drug Discovery
AI is accelerating the process of drug discovery by screening molecular structures, forecasting how drugs will behave, and discovering new drugs. Drug manufacturers are employing AI to accelerate and reduce the expense of bringing new drugs to market. DeepMind’s AlphaFold, for instance, has revolutionized how we can forecast protein structures, giving rise to new treatments and better medicine.
20. Federated Learning
Federated learning is becoming a common method in AI that maintains the privacy of data during processing. The method allows machine learning models to learn from various devices or clusters without the need to share the actual data, which improves privacy and security. It can be used in multiple fields like customized medicine and anti-fraud activities, where the security of data is extremely critical.
Industry Applications of Data Science
Data science continues to revolutionize various industries, including:
Industry | Applications |
---|---|
Healthcare | Artificial intelligence-based diagnostics, drug discovery, and patient data analysis. |
Energy | Predictive analytics for energy grid optimization and consumption. |
Business | Market trend analysis, customer insights, and process optimization. |
Transportation & Logistics | Predictive maintenance, route optimization, and demand forecasting. |
Finance | Fraud detection, algorithmic trading, and risk management. |
Marketing & Advertising | Customer segmentation, targeted advertising, and campaign optimization. |
Education | Individualized learning experience and student performance insights. |
Telecommunication | Network optimization and churn forecasting. |
Internet of Things (IoT) | Smart city planning, traffic pattern forecasting, and sensor data analysis. |
Agriculture | Precision agriculture, crop yield forecasting, and automated irrigation. |
Social Media | Influencer detection, trend forecasting, and user behavior insights. |
Natural Language Processing (NLP) | Speech recognition, chatbots, and AI-driven content creation. |
Conclusion
The future of data science is changing quickly, with innovations like AI, automation, and big data informing industries. When companies apply these emerging technologies, they will learn new methods of working and producing new ideas, illustrating how imperative data science is in today’s technology and decision-making.
Frequently Asked Questions (FAQs)
Q1: What Are The Top Data Science Trends To Look Out For In 2025?
Some of the significant trends of data science in 2025 are Augmented Analytics, AI-as-a-Service (AIaaS), Automated Machine Learning (AutoML), Data Democratization, Responsible AI, Generative AI, and advancements in Quantum Computing. One must keep himself informed about these trends to pursue a course in data science.
Q2: What Impact Will Ai Have On Data Science In 2025?
AI is transforming data science by making analysis automatic, enhancing predictions, and assisting individuals in making data-driven decisions. If you are learning data science online, you are likely to come across AI’s role in contemporary analytics.
Q3: What Is Augmented Analytics, And Why Should I Care?
Augmented Analytics applies AI and ML to assist in preparing data, discovering insights, and producing visuals automatically. This simplifies data analysis for individuals who are not data experts. Data science courses increasingly educate on Augmented Analytics so that professionals can leverage AI-powered insights.
Q4: What Makes Automl Easy To Use?
AutoML allows us to automate the process of how we select, train, and tune machine learning models. It makes it easy to apply machine learning to everyday life for new people. If you are learning data science online, AutoML will likely be a key topic.
Q5: What Is Data Science’s Contribution To Cloud Computing?
Cloud computing allows elastic data storage, robust analysis, and AI model deployment. Most data science courses emphasize cloud data science tools like AWS, Google Cloud, and Microsoft Azure.
Q6: Why Is Responsible AI Becoming Significant?
Responsible AI is assured by ethical AI development, which promotes fairness, transparency, and unbiased models. While studying data science online, you will be instructed on how to use AI responsibly.
Q7: How Do Businesses Gain From Data Democratization?
The democratization of data enables non-technical staff to analyze and get access to data, which boosts decision-making speed and innovation. The majority of today’s data science training centers on enabling business users to make data accessible.
Q8: What Is The Effect Of Quantum Computing On Data Science?
Quantum Computing can process enormous data faster than regular computers, transforming optimization, cryptography, and AI training. More advanced data science classes may include quantum computing.
Q9: How Does Generative AI Support Data Science?
Generative AI is used in content generation, data augmentation, and synthetic data. If you are studying data science in an online course, you will probably be taught its usage in deep learning and NLP.
Q10: Which Sectors Are Deriving The Maximum Benefits From These Data Science Trends?
Healthcare, finance, e-commerce, telecommunications, and cybersecurity are a few sectors that are implementing data science innovations. An industry-specific application will be part of an organized data science course to make professionals competitive.