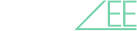
Prepzee's Cloud Masters program changed my career from SysAdmin to Cloud Expert in just 6 months. Thanks to dedicated mentors, I now excel in AWS, Terraform, Ansible, and Python.
Great learning experience through the platform. The curriculum is updated and covers all the topics. The trainers are experts in their respective fields and follow more of a practical approach.
Nice experience, I will recommend it to all the learners who are willing to join and learn IT skills. I was able to switch my domain from non-IT to IT in a reputed MNC
You're a fresher eager to start a career in Generative AI & ML using Python, machine learning,Deep learning And Gen AI
You're an IT professional aiming to transition into Generative AI & ML, with a focus on data-driven insights, predictive modeling, and AI.
You’re looking to transition into AI and Machine Learning, leveraging practical, real-world tools and applications.
You’re a professional aiming to enhance your expertise in AI and explore roles in advanced analytics, machine learning, and AI-driven decision-making.
Including Top Tools of AI/ML according to Linkedin Jobs
Learn by doing multiple labs in your learning journey.
Get a feel of AI/Ml professionals by doing real-time projects
Call us, E-Mail us whenever you stuck.
Instructors are Microsoft Certified Trainers.
Attend multiple batches until you achieve your Dream Goal.
Introduction to Python
Working with Data Structures
Working with Files
Introduction to Pandas for Data Analysis
Data Cleaning and Transformation
Exploratory Data Analysis (EDA)
Data Visualization with Matplotlib & Seaborn
Introduction to Databases with SQL Alchemy
Introduction to Data Wrangling with Numpy
Project – Amazon E-Commerce Customer Segmentation and Sales Analysis Using Python
Univariate Analysis
Bi Variate Analysis
Multi Variate Analysis
Central Tendencies
Mean, Median, Mode, Variance
Standard Deviation, Distribution
Probability Distribution
Normal Distribution
Hypothesis Testing – Inferential Statistics
What is ML
Why ML
Limitations of ML
Types of ML
– Regression
– Classification
– Clustering
– Factor Analysis
– ARIMA Modeling
Project – Sales Prediction | Stock Price Prediction
What is Deep learning
Difference between ML Vs DL Vs AI
– Pytorch | Keras | Tensorflow
– ANN | RNN | GNN | LSTM | CNN
– NLTK
– YOLO
Generative AI Models – GPT 4, DALL E, BERT
Tools & Frameworks – Hugging Face, Langchain, Open AI api
Introduction to Generative AI
What are Large Language Models (LLMs)?
Understand RNNs, their application, and limitations
What are Transformers?
Understand Attention Mechanism and Transformer Architecture
What are tokenizers?
What are embeddings?
Generating text and summarizing dialogues using LLMs
What is Prompt Engineering?
Learn optimization techniques like Prompt Engineering
Fine-tune LLMs for improved performance on tasks
Understand RLHF for improved model output.
Retrieval Augmented Generation (RAG) for knowledge grounding
LangChain essentials (chains, agents, memory)
Understanding MLOps Concepts
MLOps vs. DevOps
Proficiency in SDLC Methodologies
Basics of Cloud Computing
AWS Introduction
Version Control System
Git Common Commands
Branching and Merging
Tracking and managing changes to code
Packaging ML Models
Building CI/CD Pipelines for ML Models
AWS CodeCommit, AWS CodePipeline, AWS CodeBuild, AWS CodeDeploy
Machine Learning Model Management
Docker and Kubernetes for ML Deployment
Amazon SageMaker for ML development and deployment
Building an end-to-end MLOps pipeline using AWS SageMaker
Specializes in designing and implementing machine learning algorithms, deploying models, and optimizing them for production environments.
Builds and deploys AI models that generate content like text, images, or code using tools like GPT and other LLMs.
Designs and builds AI models to solve real-world problems using machine learning, deep learning, and Generative AI & ML techniques.
Works on neural networks and deep learning models for tasks like image processing, speech recognition, and advanced analytics
Core responsibilities include analyzing large datasets, creating machine learning models, and uncovering insights to drive business decision
Develops models to analyze and generate language-based data, with TensorFlow for advanced NLP tasks.
Online classroom pass
Embark on your journey toward a thriving career with the Generative AI and ML Job Oriented Program, designed to equip you with in-demand skills and real-world experience in the fast-evolving AI landscape. This comprehensive program covers essential and advanced tools such as Python, TensorFlow, PyTorch, MLOps, and core Machine Learning techniques, along with cutting-edge Generative AI technologies like GPT, DALL·E, BERT, and LangChain.
You’ll work on industry-relevant projects, receive expert mentorship, and get personalized support to refine your CV, strengthen your LinkedIn profile, and confidently prepare for AI and ML job opportunities.
1.1 Python Basics: Syntax, Variables, Data Types
1.2 Input and Output (print(), input())
1.3 Basic Operators and Expressions
1.4 Conditional Statements (if, elif, else)
1.5 Loops (for, while)
1.6 Data Structures: Lists, Tuples, Sets, Dictionaries
1.7 List and Dictionary Comprehensions
1.8 Iterating over Data Structures
Hands-On:
2.1 File Handling: Reading and Writing Files (Text and CSV)
2.2 Parsing CSV Files with the csv Module
2.3 Error Handling with Try/Except Blocks
2.4 Introduction to Numpy Arrays and Array Operations
2.5 Reshaping, Indexing, and Slicing Numpy Arrays
2.6 Applying Mathematical Functions and Aggregations to Numpy Arrays
2.7 Working with Multi-Dimensional Data
Hands-On:
3.1 Introduction to Pandas DataFrames and Series
3.2 Loading Data from CSV, Excel, or SQL Databases
3.3 Basic Data Frame Operations (Selection, Filtering, Sorting, Grouping)
3.4 Handling Missing Data
3.5 Data Cleaning Techniques (Dealing with Missing Data, Duplicates, Outliers)
3.6 Data Transformation: Renaming Columns, Changing Data Types, Applying Functions
3.7 Handling Dates and Times with pd.to_datetime()
3.8 Combining and Merging DataFrames
Hands-On:
4.1 Descriptive Statistics with Pandas (mean(), median(), mode(), std())
4.2 Data Visualization using Matplotlib and Seaborn (Bar Plots, Histograms, Box Plots)
4.3 Correlation Analysis and Pairwise Relationships
4.4 Detecting and Visualizing Outliers
4.5 Advanced Visualization Techniques with Matplotlib and Seaborn
4.6 Line Plots, Scatter Plots, and Pie Charts
4.7 Customizing Plots (Titles, Labels, Legends, Colors)
4.8 Creating Subplots and Multi-Panel Figures
Hands-On:
5.1 Introduction to Databases with SQLAlchemy
5.2 Writing SQL Queries from Python Using SQLAlchemy ORM
5.3 Loading Data from SQL Databases into Pandas
5.4 Writing Data Back to SQL Databases from Pandas
5.5 Introduction to Views, Stored Procedures, Functions, and Performance Optimization in Databases
Hands-On:
6.1 Univariate Analysis
6.2 Bi Variate Analysis
6.3 Multi Variate Analysis
6.4 Central Tendencies Mean, Median, Mode, Variance Standard Deviation,
6.5 Distribution Probability Distribution
6.6 Normal Distribution
6.7 Hypothesis Testing
6.8 Confidence Interval
6.9 Correlation Vs Covariance
6.10 Central Limit Theorm P value/ Alpha
6.11 Intro to Advance/ Inferential Statistics Hypothesis Testing
6.12 T test Z test Chi Square Anova
7.1 What is Machine Learning?
7.2 Why Machine Learning?
7.3 Limitations of Machine Learning
7.4 Prediction Modelling Linear Regression
7.5 Sales Predictions and Forecasting
7.6 Classification using Logistics Regression
7.7 Overview of Supervised and Unsupervised Learning
Hands-On:
8.1 Introduction to scikit-learn
8.2 Core Components of Scikit-learn for Machine learning
8.3 Understanding Regression Problems
8.4 Define regression problems
8.5 Dependent and independent variables, and their roles.
8.6 Train a Regression model using scikit-learn.
8.7 Techniques for assessing model performance
8.8 Techniques for improving model performance, such as feature scaling and polynomial regression.
8.9 Train a K-Means model on the dataset and visualize the clusters formed.
Hands-On:
9.1 Linear Regression
9.2 Logistics Regression
9.3 Decision Trees
9.4 Random Forest
9.5 Support Vector Machine (SVM)
9.6 K-Nearest Neighbors (KNN)
14.7 Time Series Forecasting
Hands-On:
10.1 K-Means Clustering
10.2 Dimensionality Reduction
10.3 Linear Discriminant Analysis (LDA)
10.4 Principal Component Analysis (PCA)
Hands-On:
11.1 Overview of Deep Learning and Neural Networks
11.2 Difference between DS VS ML VS AI
11.3 Setting up TensorFlow, Keras, and PyTorch environments
11.4 Introduction to Tensors, Computation Graphs, and Autograd
11.5 Basics of building and training models in TensorFlow and PyTorch
Hands-On:
12.1 Artificial Neural Networks (ANN)
12.2 Convolutional Neural Networks (CNN)
12.3 Recurrent Neural Networks (RNN)
12.4 Introduction to Transfer Learning
Hands-On:
13.1 Long Short-Term Memory Networks (LSTM)
13.1 Graph Neural Networks (GNN)
13.2 Advanced Recurrent Architectures: Bidirectional RNN, GRU
13.3 Hyperparameter Tuning and Model Optimization
Hands-On:
14.1 Introduction to NLP concepts and text preprocessing (tokenization, stemming)
14.2 Using NLTK for basic NLP tasks
14.3 Text classification using deep learning (RNN, LSTM)
14.4 Sentiment analysis and text generation
Hands-On:
15.1 Advanced CNNs and image processing techniques
15.2 Object detection and YOLO (You Only Look Once) for real-time detection
15.3 Transfer Learning with pre-trained image models (e.g., ResNet, Inception)
15.4 Fine-tuning models for specific tasks
Hands-On:
16.1 What is Generative AI?
16.2 Difference between Discriminative vs. Generative models
16.3 Use cases: Chatbots, Image Generation, Code Assistants
16.4 Overview of Text, Image, Audio, and Video generation
17.1 What are Large Language Models (LLMs)?
17.2 Transformer architecture & attention mechanism
17.3 Tokenization, embeddings, context windows
17.4 Pretraining vs. Fine-tuning vs. In-context learning
17.5 Zero-shot, few-shot, and chain-of-thought prompting
17.6 Prompt design best practices
17.7 Prompt debugging
Hands-On:
18.1 OpenAI API (ChatGPT, DALL·E, Whisper)
18.2 Hugging Face Inference API
18.3 Building apps using LLM APIs
19.1 LangChain essentials (chains, agents, memory)
19.2 Working with vector stores (Pinecone, FAISS, Chroma)
19.3 Retrieval-Augmented Generation (RAG)
19.4 Connecting LLMs to PDFs, websites, and SQL
20.1 Introduction to LoRA, PEFT, and parameter-efficient fine-tuning
20.2 Fine-tune small open-source models on your own data
20.3 Use cases: domain-specific chatbots, customer service models
Hosting on Hugging Face Spaces, Streamlit Cloud, or Vercel
Handling tokens, secrets, and error logging
UI/UX best practices for GenAI apps
22.1 Introduction to MLOps
22.2 MLOps vs. DevOps
22.3 SDLC Basics
22.4 What is Cloud Computing
22.5 AWS Introduction
22.6 S3
22.7 Waterfall vs AGILE vs DevOps vs MLOps
22.8 MLOps Phases
22.9 Model Packaging
23.1 Git Essentials
23.2 Configuring Git
23.3 Branching
23.4 Git Workflow
23.5 Repo
23.6 Git Commands
23.7 Tracking and managing changes to code
23.8 Source Code Management
23.9 Tracking and Saving Changes in Files
24.1 Introduction to CI/CD
24.2 CI/CD Challenges
24.3 CI/CD Implementation in ML
24.4 Popular DevOps Tools
24.5 AWS CodeCommit
24.6 AWS CodePipeline
24.7 AWS CodeBuild
24.8 AWS CodeDeploy
25.1 Docker Architecture
25.2 Docker for Machine Learning
25.3 Continuous Deployment
25.4 Writing a Dockerfile to Create an Image
25.5 Installing Docker Compose
25.6 Configuring Local Registry
25.7 Container Orchestration
25.8 Application Deployment
25.9 Kubernetes Core Concepts
26.1: CV Preperation
26.2: Interview Preperation
26.3: LinkedIn Profile Update
26.4: Expert Tips & Tricks
Our tutors are real business practitioners who hand-picked and created assignments and projects for you that you will encounter in real work.
In this LLM Project you will learn to build a knowledge-grounded chatbot using LLM's and learn how to fine tune it.
This Langchain project aims to seamlessly integrate LLM technology with databases, PDF knowledge bases, and audio processing agents to create a comprehensive customer support application.
In this AI Resume Analyzer project, you will learn to build and deploy AI resume analyzer that helps job seekers assess how effectively their resumes match job descriptions using OpenAI's language models and Azure's cloud infrastructure.
Build a personalized recommendation system for an e-commerce platform using TensorFlow. The goal is to enhance user engagement by suggesting relevant products based on customer browsing and purchase history, thus improving conversion rates and customer satisfaction.
Create a sentiment analysis and trend prediction model with PyTorch to analyze social media posts. The project will identify user sentiment, track trending topics, and predict sentiment shifts over time, supporting content moderation, marketing, and engagement strategies.
Create a stock price monitoring and alert system in Python that tracks the prices of selected stocks, analyzes trends, and sends notifications when certain price thresholds are met. This project supports informed decision-making for investors by providing instant updates and insights.
Develop a fraud detection system for e-commerce transactions using machine learning. This project aims to identify and flag potentially fraudulent transactions as they happen, helping reduce financial losses and increase customer trust.
Build an end-to-end MLOps pipeline for a customer churn prediction model in a subscription-based business. Use tools like AWS SageMaker, Docker, Kubernetes, and CI/CD pipelines to automate the deployment, monitoring, and retraining of the model.
Enrolling in the AWS Data Engineer Job Oriented Program by Prepzee for the AWS Data Engineer certification (DEA C01) was transformative. The curriculum covered critical tools like PySpark, Python, Airflow, Kafka, and Snowflake, offering a complete understanding of cloud data engineering. The hands-on labs solidified my skills, making complex concepts easy to grasp. With a perfect balance between theory and practice, I now feel confident in applying these technologies in real-world projects. Prepzee's focus on industry-relevant education was invaluable, and I’m grateful for the expertise gained from industry professionals
I enrolled in the DevOps Program at Prepzee with a focus on tools like Kubernetes, Terraform, Git, and Jenkins. This comprehensive course provided valuable resources and hands-on labs, enabling me to efficiently manage my DevOps projects. The insights gained were instrumental in leading my team and streamlining workflows. The program's balance between theory and practice enhanced my understanding of these critical tools. Additionally, the support team’s responsiveness made the learning experience smooth and enjoyable. I highly recommend the DevOps Program for anyone aiming to master these essential technologies.
Enrolling in the Data Engineer Job Oriented Program at Prepzee,, exceeded my expectations. The course materials were insightful and provided a clear roadmap for mastering these tools. The instructors' expertise and interactive learning elements made complex concepts easy to grasp. This program has been invaluable for my professional growth, giving me the confidence to apply these technologies effectively in real-world projects.
Enrolling in the Data Analyst Course for beginners at Prepzee, covering Python, SQL, Advanced Excel, and Power BI, was exactly what I needed for my career. The course content was well-structured and comprehensive, catering to both beginners and experienced learners. The hands-on labs helped reinforce key concepts, while the Prepzee team’s support was outstanding, always responsive and ready to help resolve any issues.
Prepzee has been a great partner for us and is committed towards upskilling our employee.Their catalog of training content covers a wide range of digital domains and functions, which we appreciate.The best part was there LMS on which videos were posted online for you to review if you missed anything during the class.I would recommend Prepzee to all to boost his/her learning.The trainer was also very knowledgeable and ready to answer individual questions.
Get Certified after completing this course with Prepzee